AI in Observability: A Trip or a Trap?
Generative AI or Generative Artificial Intelligence, in its simplest form, means being capable of generating text, images, or any data using generative models, mostly in response to prompts. You would have all heard of OpenAI’s ChatGPT. It is generative AI in action.
Essentially, What do you do in ChatGPT?
You type in a topic or a question, and the robot replies with structured answers. It is able to answer all your questions because it analyses them and finds relevant information from the data it has already been fed and trained on. Gen AI models learn the patterns and structure of their input training data and generate new data with similar characteristics.
ChatGPT is probably the most used word in the last few months. It has taken the world by a storm, and people involved in IT are already looking at ways to leverage this massive, powerful innovation to work the best for them.
In this article, we explore the intersection of generative AI and observability. Observability is something we all can never compromise because it is the backbone of any successfully running platform. We delve into the promises and possibilities that this convergence brings, examining how generative AI techniques such as GANs, VAEs, and DRL can augment traditional observability practices.
Moreover, we confront the ethical considerations and challenges accompanying generative AI integration into observability frameworks. As we harness the power of AI to unlock new realms of understanding and prediction, we must tread carefully, mindful of issues such as data privacy, algorithmic bias, and the potential for unintended consequences.
Table Of Contents:
- What is Generative AI?
- Challenges in Traditional Observability
- How has AI Forayed into Observability?
- Potential Applications of Generative AI in Observability
- Challenges and Considerations for Adoption
- How does the Future Look?
What is Generative AI?
Generative AI represents a frontier where machines not only analyze data but also generate it, creating synthetic data points that mimic the characteristics of real-world observations. This groundbreaking approach holds the potential to revolutionize how we perceive, monitor, and optimize systems, offering unprecedented insights and capabilities.
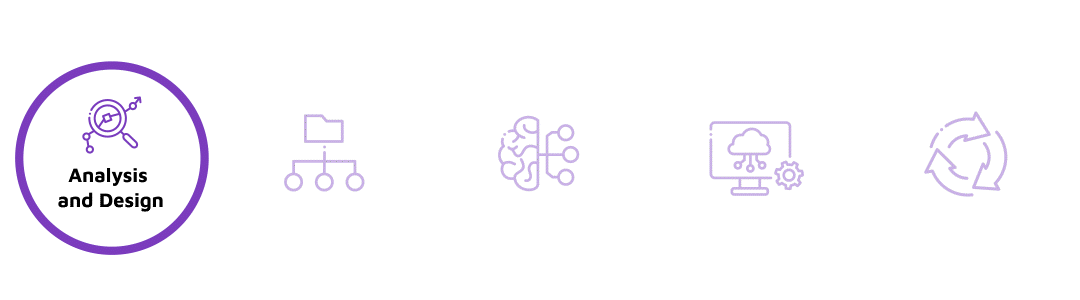
What goes into making a Gen AI Tool?
A lot of consideration goes into crafting a Gen AI tool. First and foremost is the OBJECTIVE. What is the purpose of your tool? Are you building it to create texts, images, or something else? After you have figured out the objective comes choosing an apt generative model - it can be Variational Autoencoders (VAEs), Generative Adversarial Networks (GANs), or autoregressive models like the GPT series.
(We will save the discussion on what these exactly mean for later; for now, kindly go through the links)
Once sorted with the generative model, we can train our tool on multitudes of datasets. After training, you deploy and integrate the tool into your workflows and see how it performs. Analyze and fine-tune the hyperparameters.
Challenges in Traditional Observability
Traditional observability, especially in complex systems like software applications or distributed networks, faces several challenges that can hinder the ability to effectively monitor, debug, and optimize these systems.
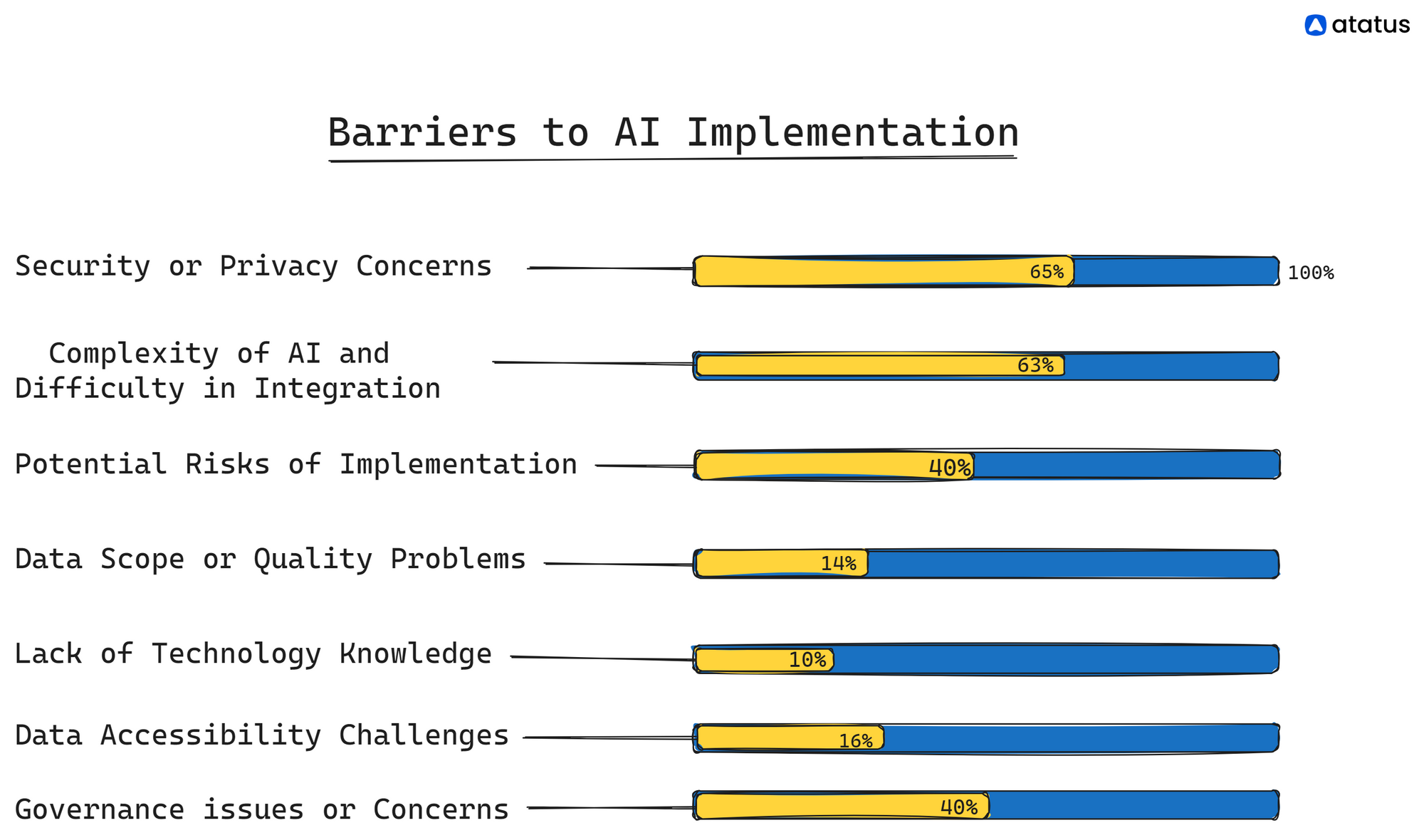
Some of the key challenges include:
- Limited Visibility - Traditional observability tools often provide limited visibility into the inner workings of complex systems. They may lack the ability to capture detailed insights into the interactions between different components, making it difficult to diagnose issues or understand performance bottlenecks.
- Silos and Fragmentation - Observability data is often fragmented across various tools and systems, leading to siloed information. This fragmentation can make it challenging to correlate data from different sources and gain a holistic view of system behavior.
- High Dimensionality - Modern systems generate vast data, achieving high-dimensional observability metrics. Managing and analyzing this data at scale can be overwhelming, leading to information overload and difficulty in extracting meaningful insights.
- Complexity and Dynamism - Systems are becoming increasingly complex and dynamic, with components deployed across diverse environments such as on-premises, cloud, and hybrid infrastructures. Traditional observability tools may struggle to keep pace with this complexity and adapt to real-time changes.
- Latency and Overhead - Some observability techniques, such as instrumentation and logging, can introduce latency and overhead into the system. This can impact the monitored system's performance and lead to inaccuracies in observability data.
- Noise and False Positives - Observability data often contains noise and false positives, which can obscure genuine issues and lead to wasted time and resources in investigating false alarms.
- Dependency on Instrumentation - Traditional observability approaches often rely on manual instrumentation of code or infrastructure components to collect data. This process can be time-consuming, error-prone, and may not capture all relevant information.
- Lack of Context - Observability data is most useful when accompanied by contextual information about the system's state, environment, and historical behavior. Traditional tools may struggle to provide this context, making it challenging to interpret observability data accurately.
Addressing these challenges requires adopting more advanced observability techniques and tools that leverage machine learning, distributed tracing, and real-time analytics.
How has AI forayed into Observability?
If you look at areas where AI has been used in the past, all of these had to do with large datasets. And precisely, it is very difficult to handle such large amounts of data manually or with simple systems. What generative AI does is not only run all that data but also scourge through it to pick out patterns and cycles. And if anything seems to deviate from the normal, they alert you.
See how easy that sounded! Well, Gen AI is created to work this and more.
Some of the areas where AI has already been implemented are:
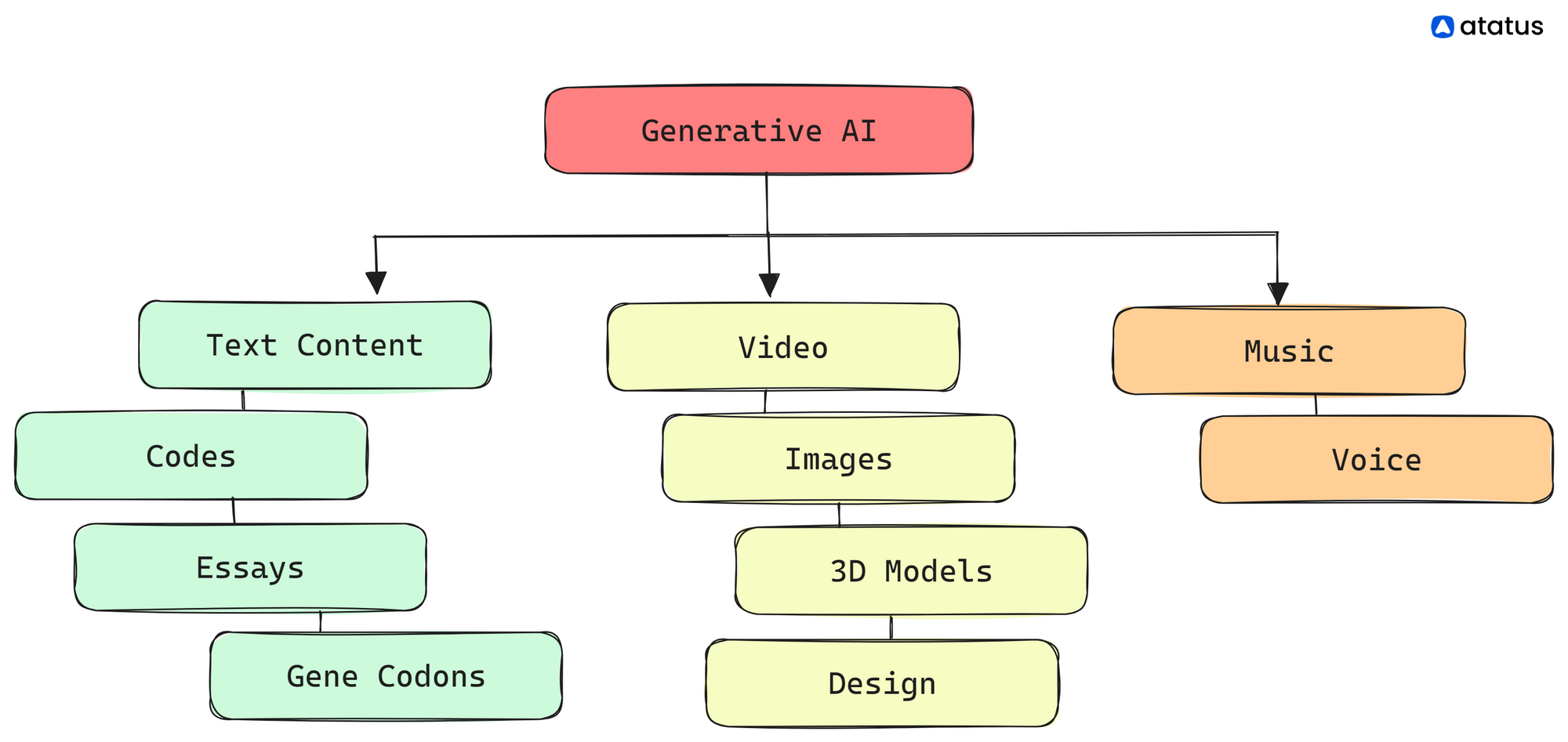
i.) Data Augmentation: Generative AI techniques have been employed to augment training datasets by generating synthetic data samples, thereby improving the robustness and generalization of machine learning models.
ii.) Predictive Insights: AI-powered predictive analytics harness historical observability data to forecast future system behaviors and trends. This capability allows organizations to proactively address impending issues before they escalate, optimizing resource allocation and minimizing downtime or performance degradation.
iii.) Natural Language Processing (NLP) Capabilities: AI-powered observability tools leverage NLP to analyze unstructured data sources such as logs and alerts. By extracting insights and facilitating human-machine interaction, these tools enhance the interpretability of observability data, enabling faster decision-making and action.
iv.) Automated Remediation: AI-powered observability platforms can automate the remediation of common issues or incidents in real time. By integrating with orchestration tools and decision-making systems, these platforms can autonomously execute predefined actions, such as scaling resources, restarting services, or rerouting traffic, to resolve problems and maintain system stability.
v.) Product Design and Simulation: Generative AI models have been utilized in product design and simulation applications to generate and explore design alternatives, optimize product performance, and simulate real-world scenarios.
AI-powered observability platforms continuously learn from past observations and feedback to refine their models, algorithms, and recommendations. These platforms can deliver increasingly accurate insights by iteratively adapting to changing environments and user requirements.
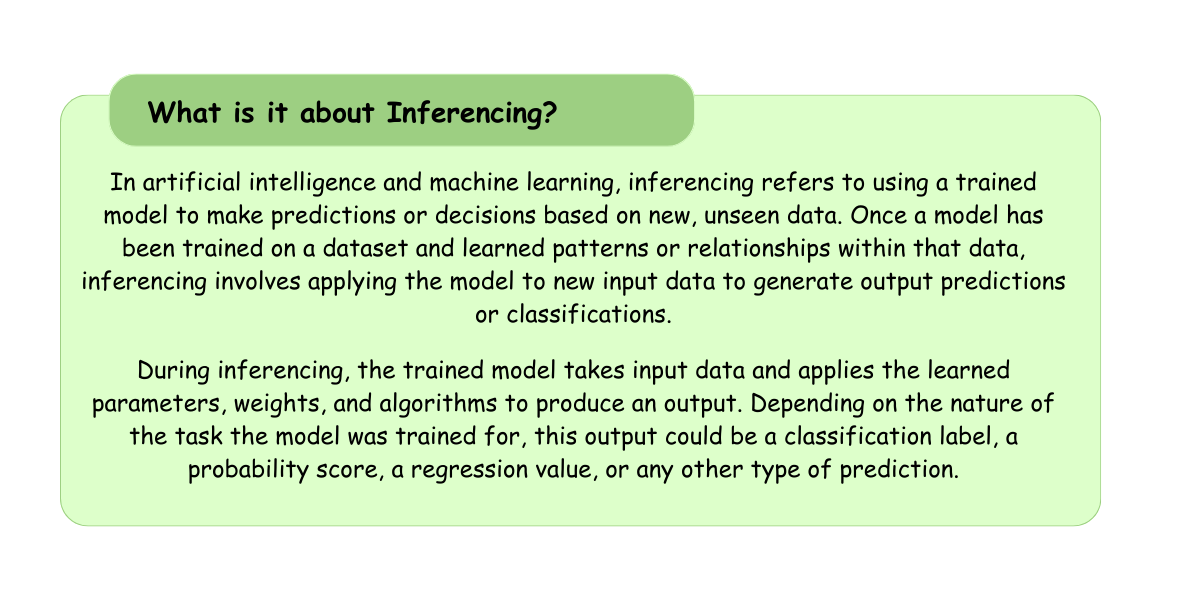
Potential Applications of Generative AI in Observability
Currently, every other technology company that has adopted AI in some form allocates only 1% of its total spending on AI technology. They combined spend close to $40 billion. But Bloomberg Intelligence says that by 2032 (i.e., within the next 8 years), this number will go up to $1.3 trillion, whereby each would spend close to 10% of their expenditure on AI-driven tools.
The reason for such futuristic prediction doesn’t stem from futility. They are part of careful considerations of how AI is interfering with and changing our working conditions.
Observability has been a game changer for many organizations (even smaller ones adopting it more frequently) in the past few years. It has saved so much for these companies that they now no longer consider buying an observability tool an additional cost but more of an investment to save a lot on other decremental problems later.
You have seen how AI has already been incorporated into traditional observability. But, the potential applications of Generative AI don’t stop there. There are many emerging techniques and scenarios where Gen AI could be of more help.
i.) Synthetic Data Generation for Testing
Generative AI could create synthetic data that simulates various system states, user interactions, and environmental conditions for testing observability tools and systems. This synthetic data could help ensure robustness and effectiveness in diverse scenarios.
ii.) Scenario Generation for Simulation
Generative AI models could generate realistic scenarios or simulations of complex system behaviors and events. These simulations could be used to evaluate the performance of observability systems under different conditions, enabling proactive optimization and resilience planning.
iii.) Automatic Documentation Generation
Generative AI could assist in automatically generating documentation, reports, or summaries based on observability data. By analyzing logs, metrics, and other observability sources, AI models could generate descriptive narratives or visualizations that provide insights into system behavior and performance trends.
iv.) Adaptive Visualization Techniques
Generative AI could be leveraged to develop adaptive visualization techniques that dynamically adjust based on the observed data characteristics. These techniques could enhance the interpretability and usability of observability dashboards, enabling operators to gain deeper insights and identify trends more effectively.
v.) Multi-modal Data Fusion and Analysis
Generative AI techniques enable the fusion and analysis of multi-modal observability data, including text logs, time-series metrics, and graphical visualizations. By synthesizing insights from diverse data sources, generative models can uncover hidden correlations, patterns, and anomalies that may not be apparent through individual data streams alone.
vi.) Interactive Chatbot Interfaces for Observability Insights
Generative AI-powered chatbot interfaces can provide conversational access to observability insights and recommendations. These chatbots can interpret natural language queries, provide contextual explanations, and offer actionable guidance to operators, enabling seamless interaction and decision-making based on observability data.
Healthcare | Ecommerce | Education | Entertainment | Utilities | Travel & Transport | Manufacturing, Supply, etc | |
---|---|---|---|---|---|---|---|
Design and Development | |||||||
Content creation | |||||||
Data Analytics | |||||||
Predictive Maintenance | |||||||
Risk Mitigation | |||||||
Chatbots and VAs |
Challenges and Considerations for Adoption
Adapting generative AI presents several challenges and considerations alongside notable use cases. The challenges include these listed below:
#1 Data Quality and Quantity
Generative AI models require large amounts of high-quality data for effective training. Ensuring the availability of diverse and representative datasets can be challenging, especially in domains with limited data or complex, unstructured data types.
#2 Ethical and Bias Concerns
Generative AI models can potentially perpetuate or amplify biases present in the training data. Ethical considerations surrounding fairness, transparency, and accountability must be carefully addressed to mitigate the risk of biased outcomes and unintended consequences.
#3 Model Complexity and Interpretability
Generative AI models are often complex and opaque, making understanding how they generate outputs or interpret their decisions challenging. Ensuring model interpretability and transparency is crucial for building trust and facilitating human oversight and intervention when necessary.
#4 Resource Intensiveness
Training and deploying generative AI models can be computationally intensive and resource-demanding, requiring specialized hardware and infrastructure. Managing costs, scalability, and performance optimization are important considerations for successful adoption.
#5 Security and Privacy Risks
Generative AI models have the potential to generate synthetic data that could compromise individual privacy or expose sensitive information. Implementing robust security measures, data anonymization techniques, and compliance with regulatory frameworks are essential to safeguarding data privacy and confidentiality.
And that is why, before adopting generative AI, organizations should:
- Understand the Use Case - Clearly define the use case and objectives for adopting generative AI, ensuring alignment with business goals and user needs.
- Evaluate Technical Feasibility - Assess the technical feasibility of implementing generative AI, considering data availability, model complexity, and computational resources.
- Address Ethical Implications - Consider the ethical implications of generative AI, including potential biases, privacy concerns, and societal impacts, and develop strategies to mitigate risks.
- Ensure Stakeholder Buy-In - Obtain buy-in from stakeholders across the organization, including management, legal, compliance, and end-users, to ensure support and alignment with organizational priorities.
- Plan for Deployment and Maintenance - Develop a clear deployment plan and strategy for ongoing maintenance, monitoring, and evaluation of the generative AI system to ensure long-term success and sustainability.
Taking care of these pointers will help you utilize the power of AI without worrying about security issues and concerns.
How does the Future Look?
It is no secret that Generative AI will create a whirlwind in the current technology sector. It will change how we look at and interact with tools for Observation, analytics, and Visualization.
In the world of observability, generative AI is proving to be a powerful tool for improving system performance and detecting anomalies. With its ability to continuously learn and adapt, generative AI offers a dynamic and efficient solution for monitoring complex systems. As technology advances, we can expect more innovative applications of generative AI in observability.
That said, when creating any new AI model, careful emphasis must be placed on its suitability for use without compromising its security and user privacy. As technology advances, we can only imagine the limitless possibilities that generative AI will bring.
Monitor Your Entire Application with Atatus
Atatus is a Full Stack Observability Platform that lets you review problems as if they happened in your application. Instead of guessing why errors happen or asking users for screenshots and log dumps, Atatus lets you replay the session to quickly understand what went wrong.
We offer Application Performance Monitoring, Real User Monitoring, Serverless Monitoring, Logs Monitoring, Synthetic Monitoring, Uptime Monitoring, and API Analytics. It works perfectly with any application, regardless of framework, and has plugins.
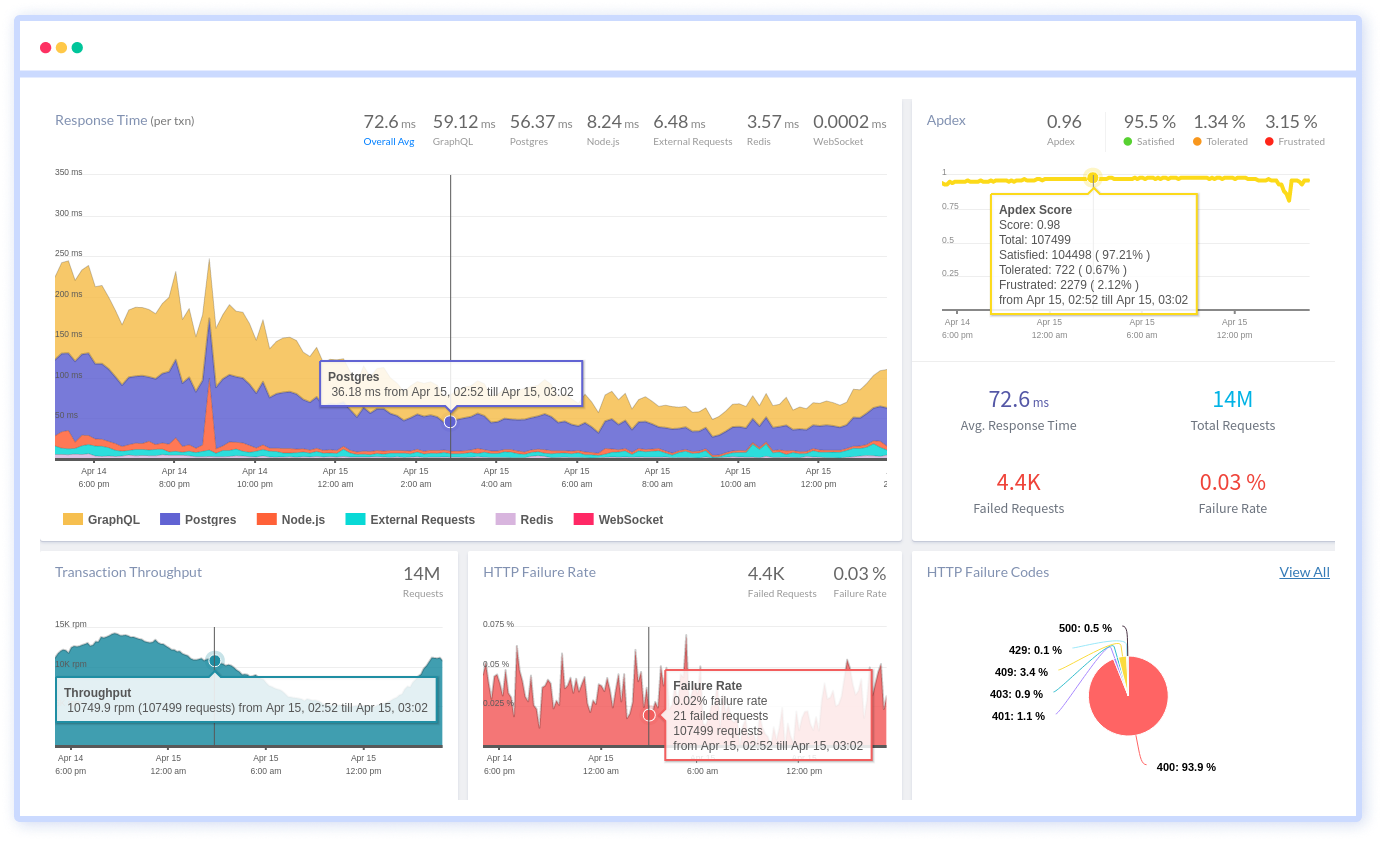
Atatus can be beneficial to your business, which provides a comprehensive view of your application, including how it works, where performance bottlenecks exist, which users are most impacted, and which errors break your code for your frontend, backend, and infrastructure.
If you are not yet an Atatus customer, you can sign up for a 14-day free trial.
#1 Solution for Logs, Traces & Metrics
APM
Kubernetes
Logs
Synthetics
RUM
Serverless
Security
More